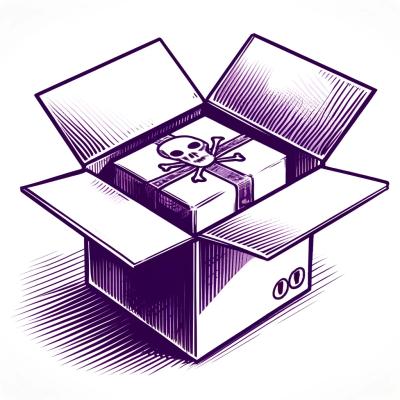
Security News
Research
Data Theft Repackaged: A Case Study in Malicious Wrapper Packages on npm
The Socket Research Team breaks down a malicious wrapper package that uses obfuscation to harvest credentials and exfiltrate sensitive data.
Fabric is the fast and lightweight way to scale PyTorch models without boilerplate
Run on any device at any scale with expert-level control over PyTorch training loop and scaling strategy. You can even write your own Trainer.
Fabric is designed for the most complex models like foundation model scaling, LLMs, diffusion, transformers, reinforcement learning, active learning. Of any size.
What to change | Resulting Fabric Code (copy me!) |
---|---|
|
|
# Use your available hardware
# no code changes needed
fabric = Fabric()
# Run on GPUs (CUDA or MPS)
fabric = Fabric(accelerator="gpu")
# 8 GPUs
fabric = Fabric(accelerator="gpu", devices=8)
# 256 GPUs, multi-node
fabric = Fabric(accelerator="gpu", devices=8, num_nodes=32)
# Run on TPUs
fabric = Fabric(accelerator="tpu")
# Use state-of-the-art distributed training techniques
fabric = Fabric(strategy="ddp")
fabric = Fabric(strategy="deepspeed")
fabric = Fabric(strategy="fsdp")
# Switch the precision
fabric = Fabric(precision="16-mixed")
fabric = Fabric(precision="64")
# no more of this!
- model.to(device)
- batch.to(device)
import lightning as L
class MyCustomTrainer:
def __init__(self, accelerator="auto", strategy="auto", devices="auto", precision="32-true"):
self.fabric = L.Fabric(accelerator=accelerator, strategy=strategy, devices=devices, precision=precision)
def fit(self, model, optimizer, dataloader, max_epochs):
self.fabric.launch()
model, optimizer = self.fabric.setup(model, optimizer)
dataloader = self.fabric.setup_dataloaders(dataloader)
model.train()
for epoch in range(max_epochs):
for batch in dataloader:
input, target = batch
optimizer.zero_grad()
output = model(input)
loss = loss_fn(output, target)
self.fabric.backward(loss)
optimizer.step()
You can find a more extensive example in our examples
Lightning is rigorously tested across multiple CPUs and GPUs and against major Python and PyTorch versions.
System / PyTorch ver. | 1.12 | 1.13 | 2.0 | 2.1 |
---|---|---|---|---|
Linux py3.9 [GPUs] | ||||
Linux (multiple Python versions) | ||||
OSX (multiple Python versions) | ||||
Windows (multiple Python versions) |
TIP: We strongly recommend creating a virtual environment first. Don’t know what this is? Follow our beginner guide here.
pip install -U lightning
Create the Fabric
object at the beginning of your training code.
import Lightning as L
fabric = L.Fabric()
Call setup()
on each model and optimizer pair and setup_dataloaders()
on all your data loaders.
model, optimizer = fabric.setup(model, optimizer)
dataloader = fabric.setup_dataloaders(dataloader)
Remove all .to
and .cuda
calls -> Fabric will take care of it.
- model.to(device)
- batch.to(device)
Replace loss.backward()
by fabric.backward(loss)
.
- loss.backward()
+ fabric.backward(loss)
Run the script from the terminal with
lightning run model path/to/train.py
or use the launch() method in a notebook. Learn more about launching distributed training.
Yes :) Fabric works with PyTorch LightningModules and Callbacks, so you can choose how to structure your code and reuse existing models and callbacks as you wish. Read more here.
If you have any questions please:
FAQs
Unknown package
We found that lightning-fabric demonstrated a healthy version release cadence and project activity because the last version was released less than a year ago. It has 2 open source maintainers collaborating on the project.
Did you know?
Socket for GitHub automatically highlights issues in each pull request and monitors the health of all your open source dependencies. Discover the contents of your packages and block harmful activity before you install or update your dependencies.
Security News
Research
The Socket Research Team breaks down a malicious wrapper package that uses obfuscation to harvest credentials and exfiltrate sensitive data.
Research
Security News
Attackers used a malicious npm package typosquatting a popular ESLint plugin to steal sensitive data, execute commands, and exploit developer systems.
Security News
The Ultralytics' PyPI Package was compromised four times in one weekend through GitHub Actions cache poisoning and failure to rotate previously compromised API tokens.