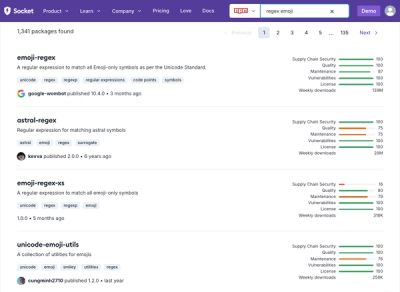
Security News
Weekly Downloads Now Available in npm Package Search Results
Socket's package search now displays weekly downloads for npm packages, helping developers quickly assess popularity and make more informed decisions.
⏲️ Easy rate limiting for Python. Rate limiting async and thread-safe decorators and context managers that use a token bucket algorithm.
limiter
makes it easy to add rate limiting to Python projects, using
a token bucket algorithm. limiter
can provide Python projects and
scripts with:
Here are some features and benefits of using limiter
:
Here's an example of using a limiter as a decorator and context manager:
from aiohttp import ClientSession
from limiter import Limiter
limit_downloads = Limiter(rate=2, capacity=5, consume=2)
@limit_downloads
async def download_image(url: str) -> bytes:
async with ClientSession() as session, session.get(url) as response:
return await response.read()
async def download_page(url: str) -> str:
async with (
ClientSession() as session,
limit_downloads,
session.get(url) as response
):
return await response.text()
You can define limiters and use them dynamically across your project.
Note: If you're using Python version 3.9.x
or below, check
out the documentation for version 0.2.0
of limiter
here.
Limiter
instancesLimiter
instances take rate
, capacity
and consume
arguments.
rate
is the token replenishment rate per second. Tokens are automatically added every second.consume
is the amount of tokens consumed from the token bucket upon successfully taking tokens from the bucket.capacity
is the total amount of tokens the token bucket can hold. Token replenishment stops when this capacity is
reached.limiter
can rate limit all Python callables, and limiters can be used as context managers.
You can define a limiter with a set refresh rate
and total token capacity
. You can set the amount of tokens to
consume dynamically with consume
, and the bucket
parameter sets the bucket to consume tokens from:
from limiter import Limiter
REFRESH_RATE: int = 2
BURST_RATE: int = 3
MSG_BUCKET: str = 'messages'
limiter: Limiter = Limiter(rate=REFRESH_RATE, capacity=BURST_RATE)
limit_msgs: Limiter = limiter(bucket=MSG_BUCKET)
@limiter
def download_page(url: str) -> bytes:
...
@limiter(consume=2)
async def download_page(url: str) -> bytes:
...
def send_page(page: bytes):
with limiter(consume=1.5, bucket=MSG_BUCKET):
...
async def send_page(page: bytes):
async with limit_msgs:
...
@limit_msgs(consume=3)
def send_email(to: str):
...
async def send_email(to: str):
async with limiter(bucket=MSG_BUCKET):
...
In the example above, both limiter
and limit_msgs
share the same limiter. The only difference is that limit_msgs
will take tokens from the MSG_BUCKET
bucket by default.
assert limiter.limiter is limit_msgs.limiter
assert limiter.bucket != limit_msgs.bucket
assert limiter != limit_msgs
You can reuse existing limiters in your code, and you can create new limiters from the parameters of an existing limiter
using the new()
method.
Or, you can define a new limiter entirely:
# you can reuse existing limiters
limit_downloads: Limiter = limiter(consume=2)
# you can use the settings from an existing limiter in a new limiter
limit_downloads: Limiter = limiter.new(consume=2)
# or you can simply define a new limiter
limit_downloads: Limiter = Limiter(REFRESH_RATE, BURST_RATE, consume=2)
@limit_downloads
def download_page(url: str) -> bytes:
...
@limit_downloads
async def download_page(url: str) -> bytes:
...
def download_image(url: str) -> bytes:
with limit_downloads:
...
async def download_image(url: str) -> bytes:
async with limit_downloads:
...
Let's look at the difference between reusing an existing limiter, and creating new limiters with the new()
method:
limiter_a: Limiter = limiter(consume=2)
limiter_b: Limiter = limiter.new(consume=2)
limiter_c: Limiter = Limiter(REFRESH_RATE, BURST_RATE, consume=2)
assert limiter_a != limiter
assert limiter_a != limiter_b != limiter_c
assert limiter_a != limiter_b
assert limiter_a.limiter is limiter.limiter
assert limiter_a.limiter is not limiter_b.limiter
assert limiter_a.attrs == limiter_b.attrs == limiter_c.attrs
The only things that are equivalent between the three new limiters above are the limiters' attributes, like
the rate
, capacity
, and consume
attributes.
You don't have to assign Limiter
objects to variables. Anonymous limiters don't share a token bucket like named
limiters can. They work well when you don't have a reason to share a limiter between two or more blocks of code, and
when a limiter has a single or independent purpose.
limiter
, after version v0.3.0
, ships with a limit
type alias for Limiter
:
from limiter import limit
@limit(capacity=2, consume=2)
async def send_message():
...
async def upload_image():
async with limit(capacity=3) as limiter:
...
The above is equivalent to the below:
from limiter import Limiter
@Limiter(capacity=2, consume=2)
async def send_message():
...
async def upload_image():
async with Limiter(capacity=3) as limiter:
...
Both limit
and Limiter
are the same object:
assert limit is Limiter
A Limiter
's jitter
argument adds jitter to help with contention.
The value is in units
, which is milliseconds by default, and can be any of these:
False
, to add no jitter. This is the default.True
, to add a random amount of jitter.range
object, to add a random amount of jitter within the range.tuple
of two numbers, start
and stop
, to add a random amount of jitter between the two numbers.tuple
of three numbers: start
, stop
and step
, to add jitter like you would with range
.For example, if you want to use a random amount of jitter between 0
and 100
milliseconds:
limiter = Limiter(rate=2, capacity=5, consume=2, jitter=(0, 100))
limiter = Limiter(rate=2, capacity=5, consume=2, jitter=(0, 100, 1))
limiter = Limiter(rate=2, capacity=5, consume=2, jitter=range(0, 100))
limiter = Limiter(rate=2, capacity=5, consume=2, jitter=range(0, 100, 1))
All of the above are equivalent to each other in function.
You can also supply values for jitter
when using decorators or context-managers:
limiter = Limiter(rate=2, capacity=5, consume=2)
@limiter(jitter=range(0, 100))
def download_page(url: str) -> bytes:
...
async def download_page(url: str) -> bytes:
async with limiter(jitter=(0, 100)):
...
You can use the above to override default values of jitter
in a Limiter
instance.
To add a small amount of random jitter, supply True
as the value:
limiter = Limiter(rate=2, capacity=5, consume=2, jitter=True)
# or
@limiter(jitter=True)
def download_page(url: str) -> bytes:
...
To turn off jitter in a Limiter
configured with jitter, you can supply False
as the value:
limiter = Limiter(rate=2, capacity=5, consume=2, jitter=range(10))
@limiter(jitter=False)
def download_page(url: str) -> bytes:
...
async def download_page(url: str) -> bytes:
async with limiter(jitter=False):
...
Or create a new limiter with jitter turned off:
limiter: Limiter = limiter.new(jitter=False)
units
is a number representing the amount of units in one second. The default value is 1000
for 1,000 milliseconds in one second.
Similar to jitter
, units
can be supplied at all the same call sites and constructors that jitter
is accepted.
If you want to use a different unit than milliseconds, supply a different value for units
.
0.3.0
and up0.3.0
$ python3 -m pip install limiter
See LICENSE
. If you'd like to use this project with a different license, please get in touch.
FAQs
⏲️ Easy rate limiting for Python. Rate limiting async and thread-safe decorators and context managers that use a token bucket algorithm.
We found that limiter demonstrated a healthy version release cadence and project activity because the last version was released less than a year ago. It has 1 open source maintainer collaborating on the project.
Did you know?
Socket for GitHub automatically highlights issues in each pull request and monitors the health of all your open source dependencies. Discover the contents of your packages and block harmful activity before you install or update your dependencies.
Security News
Socket's package search now displays weekly downloads for npm packages, helping developers quickly assess popularity and make more informed decisions.
Security News
A Stanford study reveals 9.5% of engineers contribute almost nothing, costing tech $90B annually, with remote work fueling the rise of "ghost engineers."
Research
Security News
Socket’s threat research team has detected six malicious npm packages typosquatting popular libraries to insert SSH backdoors.