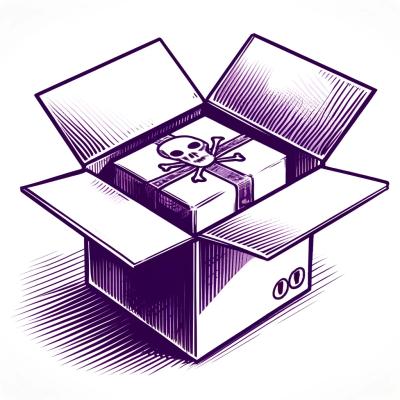
Security News
Research
Data Theft Repackaged: A Case Study in Malicious Wrapper Packages on npm
The Socket Research Team breaks down a malicious wrapper package that uses obfuscation to harvest credentials and exfiltrate sensitive data.
llama-index-llms-llama-cpp
Advanced tools
Install the required Python packages:
%pip install llama-index-embeddings-huggingface
%pip install llama-index-llms-llama-cpp
!pip install llama-index
from llama_index.core import SimpleDirectoryReader, VectorStoreIndex
from llama_index.llms.llama_cpp import LlamaCPP
from llama_index.llms.llama_cpp.llama_utils import (
messages_to_prompt,
completion_to_prompt,
)
Set up the model URL and initialize the LlamaCPP LLM:
model_url = "https://huggingface.co/TheBloke/Llama-2-13B-chat-GGML/resolve/main/llama-2-13b-chat.ggmlv3.q4_0.bin"
llm = LlamaCPP(
model_url=model_url,
temperature=0.1,
max_new_tokens=256,
context_window=3900,
generate_kwargs={},
model_kwargs={"n_gpu_layers": 1},
messages_to_prompt=messages_to_prompt,
completion_to_prompt=completion_to_prompt,
verbose=True,
)
Use the complete
method to generate a response:
response = llm.complete("Hello! Can you tell me a poem about cats and dogs?")
print(response.text)
You can also stream completions for a prompt:
response_iter = llm.stream_complete("Can you write me a poem about fast cars?")
for response in response_iter:
print(response.delta, end="", flush=True)
Change the global tokenizer to match the LLM:
from llama_index.core import set_global_tokenizer
from transformers import AutoTokenizer
set_global_tokenizer(
AutoTokenizer.from_pretrained("NousResearch/Llama-2-7b-chat-hf").encode
)
Set up the embedding model and load documents:
from llama_index.embeddings.huggingface import HuggingFaceEmbedding
embed_model = HuggingFaceEmbedding(model_name="BAAI/bge-small-en-v1.5")
documents = SimpleDirectoryReader(
"../../../examples/paul_graham_essay/data"
).load_data()
Create a vector store index from the loaded documents:
index = VectorStoreIndex.from_documents(documents, embed_model=embed_model)
Set up the query engine with the LlamaCPP LLM:
query_engine = index.as_query_engine(llm=llm)
response = query_engine.query("What did the author do growing up?")
print(response)
https://docs.llamaindex.ai/en/stable/examples/llm/llama_2_llama_cpp/
FAQs
llama-index llms llama cpp integration
We found that llama-index-llms-llama-cpp demonstrated a healthy version release cadence and project activity because the last version was released less than a year ago. It has 1 open source maintainer collaborating on the project.
Did you know?
Socket for GitHub automatically highlights issues in each pull request and monitors the health of all your open source dependencies. Discover the contents of your packages and block harmful activity before you install or update your dependencies.
Security News
Research
The Socket Research Team breaks down a malicious wrapper package that uses obfuscation to harvest credentials and exfiltrate sensitive data.
Research
Security News
Attackers used a malicious npm package typosquatting a popular ESLint plugin to steal sensitive data, execute commands, and exploit developer systems.
Security News
The Ultralytics' PyPI Package was compromised four times in one weekend through GitHub Actions cache poisoning and failure to rotate previously compromised API tokens.