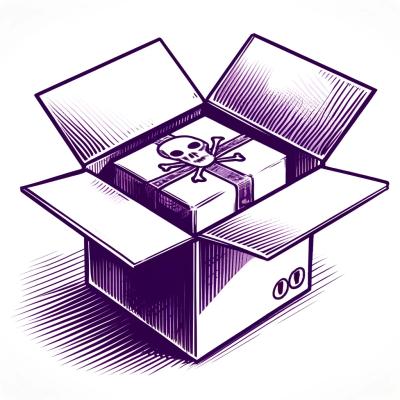
Security News
Research
Data Theft Repackaged: A Case Study in Malicious Wrapper Packages on npm
The Socket Research Team breaks down a malicious wrapper package that uses obfuscation to harvest credentials and exfiltrate sensitive data.
MegaBlocks is a light-weight library for mixture-of-experts (MoE) training. The core of the system is efficient "dropless-MoE" (dMoE, paper) and standard MoE layers.
MegaBlocks is integrated with Megatron-LM, where we support data, expert and pipeline parallel training of MoEs. Stay tuned for tighter integration with Databricks libraries and tools!
MegaBlocks dMoEs outperform MoEs trained with Tutel by up to 40% compared to Tutel's best performing capacity_factor
configuration. MegaBlocks dMoEs use a reformulation of MoEs in terms of block-sparse operations, which allows us to avoid token dropping without sacrificing hardware efficiency. In addition to being faster, MegaBlocks simplifies MoE training by removing the capacity_factor
hyperparameter altogether. Compared to dense Transformers trained with Megatron-LM, MegaBlocks dMoEs can accelerate training by as much as 2.4x. Check out our paper for more details!
NOTE: This assumes you have numpy
and torch
installed.
Training models with Megatron-LM: We recommend using NGC's nvcr.io/nvidia/pytorch:23.09-py3
PyTorch container. The Dockerfile builds on this image with additional dependencies. To build the image, run docker build . -t megablocks-dev
and then bash docker.sh
to launch the container. Once inside the container, install MegaBlocks with pip install .
. See Usage for instructions on training MoEs with MegaBlocks + Megatron-LM.
Using MegaBlocks in other packages: To install the MegaBlocks package for use in other frameworks, run pip install megablocks
. For example, Mixtral-8x7B can be run with vLLM + MegaBlocks with this installation method.
Extras: MegaBlocks has optional dependencies that enable additional features.
Installing megablocks[gg]
enables dMoE computation with grouped GEMM. This feature is enabled by setting the mlp_impl
argument to grouped
. This is currently our recommended path for Hopper-generation GPUs.
Installing megablocks[dev]
allows you to contribute to MegaBlocks and test locally. Installing megablocks[testing]
allows you to test via Github Actions. If you've installed megablocks[dev], you can run pre-commit install to configure the pre-commit hook to automatically format the code.
MegaBlocks can be installed with all dependencies (except for testing
) via the megablocks[all]
package.
We provide scripts for pre-training Transformer MoE and dMoE language models under the top-level directory. The quickest way to get started is to use one of the experiment launch scripts. These scripts require a dataset in Megatron-LM's format, which can be created by following their instructions.
@article{megablocks,
title={{MegaBlocks: Efficient Sparse Training with Mixture-of-Experts}},
author={Trevor Gale and Deepak Narayanan and Cliff Young and Matei Zaharia},
journal={Proceedings of Machine Learning and Systems},
volume={5},
year={2023}
}
FAQs
MegaBlocks
We found that megablocks demonstrated a healthy version release cadence and project activity because the last version was released less than a year ago. It has 7 open source maintainers collaborating on the project.
Did you know?
Socket for GitHub automatically highlights issues in each pull request and monitors the health of all your open source dependencies. Discover the contents of your packages and block harmful activity before you install or update your dependencies.
Security News
Research
The Socket Research Team breaks down a malicious wrapper package that uses obfuscation to harvest credentials and exfiltrate sensitive data.
Research
Security News
Attackers used a malicious npm package typosquatting a popular ESLint plugin to steal sensitive data, execute commands, and exploit developer systems.
Security News
The Ultralytics' PyPI Package was compromised four times in one weekend through GitHub Actions cache poisoning and failure to rotate previously compromised API tokens.