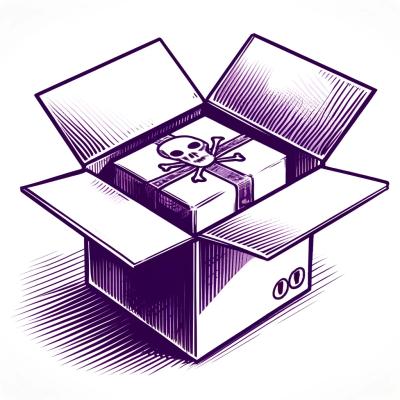
Security News
Research
Data Theft Repackaged: A Case Study in Malicious Wrapper Packages on npm
The Socket Research Team breaks down a malicious wrapper package that uses obfuscation to harvest credentials and exfiltrate sensitive data.
Pandas-plink is a Python package for reading PLINK binary file format andrealized relationship matrices (PLINK or GCTA). The file reading is taken place via lazy loading, meaning that it saves up memory by actually reading only the genotypes that are actually accessed by the user.
Notable changes can be found at the CHANGELOG.md.
It can be installed using pip:
pip install pandas-plink
Alternatively it can be intalled via conda:
conda install -c conda-forge pandas-plink
It is as simple as
>>> from pandas_plink import read_plink1_bin
>>> G = read_plink1_bin("chr11.bed", "chr11.bim", "chr11.fam", verbose=False)
>>> print(G)
<xarray.DataArray 'genotype' (sample: 14, variant: 779)>
dask.array<shape=(14, 779), dtype=float64, chunksize=(14, 779)>
Coordinates:
* sample (sample) object 'B001' 'B002' 'B003' ... 'B012' 'B013' 'B014'
* variant (variant) object '11_316849996' '11_316874359' ... '11_345698259'
father (sample) <U1 '0' '0' '0' '0' '0' '0' ... '0' '0' '0' '0' '0' '0'
fid (sample) <U4 'B001' 'B002' 'B003' 'B004' ... 'B012' 'B013' 'B014'
gender (sample) <U1 '0' '0' '0' '0' '0' '0' ... '0' '0' '0' '0' '0' '0'
i (sample) int64 0 1 2 3 4 5 6 7 8 9 10 11 12 13
iid (sample) <U4 'B001' 'B002' 'B003' 'B004' ... 'B012' 'B013' 'B014'
mother (sample) <U1 '0' '0' '0' '0' '0' '0' ... '0' '0' '0' '0' '0' '0'
trait (sample) <U2 '-9' '-9' '-9' '-9' '-9' ... '-9' '-9' '-9' '-9' '-9'
a0 (variant) <U1 'C' 'G' 'G' 'C' 'C' 'T' ... 'T' 'A' 'C' 'A' 'A' 'T'
a1 (variant) <U1 'T' 'C' 'C' 'T' 'T' 'A' ... 'C' 'G' 'T' 'G' 'C' 'C'
chrom (variant) <U2 '11' '11' '11' '11' '11' ... '11' '11' '11' '11' '11'
cm (variant) float64 0.0 0.0 0.0 0.0 0.0 0.0 ... 0.0 0.0 0.0 0.0 0.0
pos (variant) int64 157439 181802 248969 ... 28937375 28961091 29005702
snp (variant) <U9 '316849996' '316874359' ... '345653648' '345698259'
>>> print(G.sel(sample="B003", variant="11_316874359").values)
0.0
>>> print(G.a0.sel(variant="11_316874359").values)
G
>>> print(G.sel(sample="B003", variant="11_316941526").values)
2.0
>>> print(G.a1.sel(variant="11_316941526").values)
C
Portions of the genotype will be read as the user access them.
Covariance matrices can also be read very easily. Example:
>>> from pandas_plink import read_rel
>>> K = read_rel("plink2.rel.bin")
>>> print(K)
<xarray.DataArray (sample_0: 10, sample_1: 10)>
array([[ 0.885782, 0.233846, -0.186339, -0.009789, -0.138897, 0.287779,
0.269977, -0.231279, -0.095472, -0.213979],
[ 0.233846, 1.077493, -0.452858, 0.192877, -0.186027, 0.171027,
0.406056, -0.013149, -0.131477, -0.134314],
[-0.186339, -0.452858, 1.183312, -0.040948, -0.146034, -0.204510,
-0.314808, -0.042503, 0.296828, -0.011661],
[-0.009789, 0.192877, -0.040948, 0.895360, -0.068605, 0.012023,
0.057827, -0.192152, -0.089094, 0.174269],
[-0.138897, -0.186027, -0.146034, -0.068605, 1.183237, 0.085104,
-0.032974, 0.103608, 0.215769, 0.166648],
[ 0.287779, 0.171027, -0.204510, 0.012023, 0.085104, 0.956921,
0.065427, -0.043752, -0.091492, -0.227673],
[ 0.269977, 0.406056, -0.314808, 0.057827, -0.032974, 0.065427,
0.714746, -0.101254, -0.088171, -0.063964],
[-0.231279, -0.013149, -0.042503, -0.192152, 0.103608, -0.043752,
-0.101254, 1.423033, -0.298255, -0.074334],
[-0.095472, -0.131477, 0.296828, -0.089094, 0.215769, -0.091492,
-0.088171, -0.298255, 0.910274, -0.024663],
[-0.213979, -0.134314, -0.011661, 0.174269, 0.166648, -0.227673,
-0.063964, -0.074334, -0.024663, 0.914586]])
Coordinates:
* sample_0 (sample_0) object 'HG00419' 'HG00650' ... 'NA20508' 'NA20753'
* sample_1 (sample_1) object 'HG00419' 'HG00650' ... 'NA20508' 'NA20753'
fid (sample_1) object 'HG00419' 'HG00650' ... 'NA20508' 'NA20753'
iid (sample_1) object 'HG00419' 'HG00650' ... 'NA20508' 'NA20753'
>>> print(K.values)
[[ 0.89 0.23 -0.19 -0.01 -0.14 0.29 0.27 -0.23 -0.10 -0.21]
[ 0.23 1.08 -0.45 0.19 -0.19 0.17 0.41 -0.01 -0.13 -0.13]
[-0.19 -0.45 1.18 -0.04 -0.15 -0.20 -0.31 -0.04 0.30 -0.01]
[-0.01 0.19 -0.04 0.90 -0.07 0.01 0.06 -0.19 -0.09 0.17]
[-0.14 -0.19 -0.15 -0.07 1.18 0.09 -0.03 0.10 0.22 0.17]
[ 0.29 0.17 -0.20 0.01 0.09 0.96 0.07 -0.04 -0.09 -0.23]
[ 0.27 0.41 -0.31 0.06 -0.03 0.07 0.71 -0.10 -0.09 -0.06]
[-0.23 -0.01 -0.04 -0.19 0.10 -0.04 -0.10 1.42 -0.30 -0.07]
[-0.10 -0.13 0.30 -0.09 0.22 -0.09 -0.09 -0.30 0.91 -0.02]
[-0.21 -0.13 -0.01 0.17 0.17 -0.23 -0.06 -0.07 -0.02 0.91]]
Please, refer to the pandas-plink documentation for more information.
This project is licensed under the MIT License.
FAQs
Read PLINK files into Pandas data frames
We found that pandas-plink demonstrated a healthy version release cadence and project activity because the last version was released less than a year ago. It has 1 open source maintainer collaborating on the project.
Did you know?
Socket for GitHub automatically highlights issues in each pull request and monitors the health of all your open source dependencies. Discover the contents of your packages and block harmful activity before you install or update your dependencies.
Security News
Research
The Socket Research Team breaks down a malicious wrapper package that uses obfuscation to harvest credentials and exfiltrate sensitive data.
Research
Security News
Attackers used a malicious npm package typosquatting a popular ESLint plugin to steal sensitive data, execute commands, and exploit developer systems.
Security News
The Ultralytics' PyPI Package was compromised four times in one weekend through GitHub Actions cache poisoning and failure to rotate previously compromised API tokens.