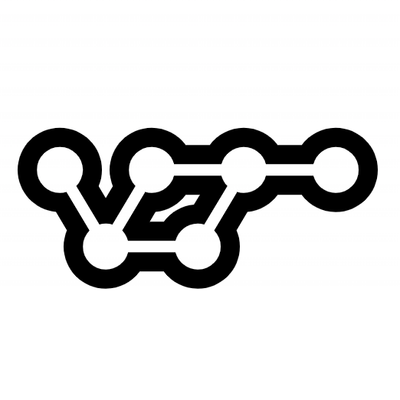
Security News
vlt Launches "reproduce": A New Tool Challenging the Limits of Package Provenance
vlt's new "reproduce" tool verifies npm packages against their source code, outperforming traditional provenance adoption in the JavaScript ecosystem.
The source code is currently hosted on GitHub at: https://github.com/jquant/jai-sdk
The latest version of JAI-SDK can be installed from pip
:
pip install jai-sdk --user
Nowadays, JAI supports python 3.7+. For more information, here is our documentation.
JAI requires an auth key to organize and secure collections. You can quickly generate your free-forever auth-key by running the command below:
from jai import get_auth_key
get_auth_key(email='email@mail.com', firstName='Jai', lastName='Z')
ATTENTION: Your auth key will be sent to your e-mail, so please make sure to use a valid address and check your spam folder.
With JAI, you can train models in the cloud and run inference on your trained models. Besides, you can achieve all your models through a REST API endpoint.
First, you can set your auth key into an environment variable or use a :file:.env
file or :file:.ini
file.
Please check the section How to configure your auth key for more information.
Bellow an example of the content of the :file:.env
file:
JAI_AUTH="xXxxxXXxXXxXXxXXxXXxXXxXXxxx"
In the below example, we'll show how to train a simple supervised model (regression) using the California housing dataset, run a prediction from this model, and call this prediction directly from the REST API.
import pandas as pd
from jai import Jai
from sklearn.datasets import fetch_california_housing
# Load dataset
data, labels = fetch_california_housing(as_frame=True, return_X_y=True)
model_data = pd.concat([data, labels], axis=1)
# Instanciating JAI class
j = Jai()
# Send data to JAI for feature extraction
j.fit(
name='california_supervised', # JAI collection name
data=model_data, # Data to be processed
db_type='Supervised', # Your training type ('Supervised', 'SelfSupervised' etc)
verbose=2,
hyperparams={
'learning_rate': 3e-4,
'pretraining_ratio': 0.8
},
label={
'task': 'regression',
'label_name': 'MedHouseVal'
},
overwrite=True)
# Run prediction
j.predict(name='california_supervised', data=data)
In this example, you could train a supervised model with the California housing dataset and run a prediction with some data.
JAI supports many other training models, like self-supervised model training. Besides, it also can train on different data types, like text and images. You can find a complete list of the model types supported by JAI on The Fit Method.
For more information, here is our documentation.
FAQs
JAI - Trust your data
We found that jai-sdk demonstrated a healthy version release cadence and project activity because the last version was released less than a year ago. It has 1 open source maintainer collaborating on the project.
Did you know?
Socket for GitHub automatically highlights issues in each pull request and monitors the health of all your open source dependencies. Discover the contents of your packages and block harmful activity before you install or update your dependencies.
Security News
vlt's new "reproduce" tool verifies npm packages against their source code, outperforming traditional provenance adoption in the JavaScript ecosystem.
Research
Security News
Socket researchers uncovered a malicious PyPI package exploiting Deezer’s API to enable coordinated music piracy through API abuse and C2 server control.
Research
The Socket Research Team discovered a malicious npm package, '@ton-wallet/create', stealing cryptocurrency wallet keys from developers and users in the TON ecosystem.