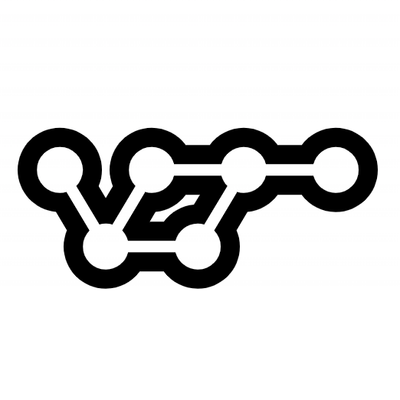
Security News
vlt Launches "reproduce": A New Tool Challenging the Limits of Package Provenance
vlt's new "reproduce" tool verifies npm packages against their source code, outperforming traditional provenance adoption in the JavaScript ecosystem.
Position embedding layers in Keras.
pip install keras-pos-embd
from tensorflow import keras
from keras_pos_embd import PositionEmbedding
model = keras.models.Sequential()
model.add(PositionEmbedding(
input_shape=(None,),
input_dim=10, # The maximum absolute value of positions.
output_dim=2, # The dimension of embeddings.
mask_zero=10000, # The index that presents padding (because `0` will be used in relative positioning).
mode=PositionEmbedding.MODE_EXPAND,
))
model.compile('adam', 'mse')
model.summary()
Note that you don't need to enable mask_zero
if you want to add/concatenate other layers like word embeddings with masks:
from tensorflow import keras
from keras_pos_embd import PositionEmbedding
model = keras.models.Sequential()
model.add(keras.layers.Embedding(
input_shape=(None,),
input_dim=10,
output_dim=5,
mask_zero=True,
))
model.add(PositionEmbedding(
input_dim=100,
output_dim=5,
mode=PositionEmbedding.MODE_ADD,
))
model.compile('adam', 'mse')
model.summary()
The sine and cosine embedding has no trainable weights. The layer has three modes, it works just like PositionEmbedding
in expand
mode:
from tensorflow import keras
from keras_pos_embd import TrigPosEmbedding
model = keras.models.Sequential()
model.add(TrigPosEmbedding(
input_shape=(None,),
output_dim=30, # The dimension of embeddings.
mode=TrigPosEmbedding.MODE_EXPAND, # Use `expand` mode
))
model.compile('adam', 'mse')
model.summary()
If you want to add this embedding to existed embedding, then there is no need to add a position input in add
mode:
from tensorflow import keras
from keras_pos_embd import TrigPosEmbedding
model = keras.models.Sequential()
model.add(keras.layers.Embedding(
input_shape=(None,),
input_dim=10,
output_dim=5,
mask_zero=True,
))
model.add(TrigPosEmbedding(
output_dim=5,
mode=TrigPosEmbedding.MODE_ADD,
))
model.compile('adam', 'mse')
model.summary()
FAQs
Position embedding layers in Keras
We found that keras-pos-embd demonstrated a healthy version release cadence and project activity because the last version was released less than a year ago. It has 1 open source maintainer collaborating on the project.
Did you know?
Socket for GitHub automatically highlights issues in each pull request and monitors the health of all your open source dependencies. Discover the contents of your packages and block harmful activity before you install or update your dependencies.
Security News
vlt's new "reproduce" tool verifies npm packages against their source code, outperforming traditional provenance adoption in the JavaScript ecosystem.
Research
Security News
Socket researchers uncovered a malicious PyPI package exploiting Deezer’s API to enable coordinated music piracy through API abuse and C2 server control.
Research
The Socket Research Team discovered a malicious npm package, '@ton-wallet/create', stealing cryptocurrency wallet keys from developers and users in the TON ecosystem.