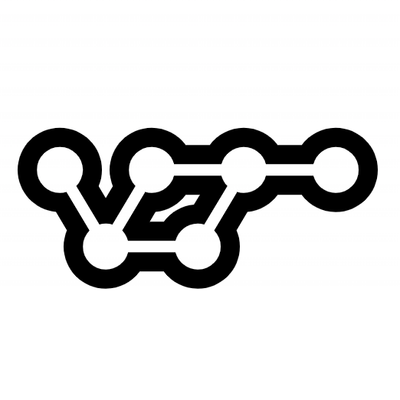
Security News
vlt Launches "reproduce": A New Tool Challenging the Limits of Package Provenance
vlt's new "reproduce" tool verifies npm packages against their source code, outperforming traditional provenance adoption in the JavaScript ecosystem.
An API to measure evaluation criteria (ex: faithfulness) of generative AI outputs
Library of tools to evaluate your RAG system.
pip install lastmile-eval
To get a LastMile AI token, please go to the LastMile token's webpage. You can create an account with Google or Github and then click the "Create new token" in the "API Tokens" section. Once a token is created, be sure to save it somewhere since you won't be able to see the value of it from the website again (though you can create a new one if that happens).
Please be careful not to share your token on GitHub. Instead we recommend saving it under your project’s (or home directory) .env
file as: LASTMILE_API_TOKEN=<TOKEN_HERE>
, and use dotenv.load_dotenv()
instead. See the examples/
folder for how to do this.
.env
file)In order to use LLM-based evaluators, add your other API tokens to your .env file.
Example: OPENAI_API_KEY=<TOKEN_HERE>
"""The RAG evaluation API runs evaluation criteria (ex: faithfulness)
of generative AI outputs from a RAG system.
Particularly, we evaluate based on this triplet of information:
1. User query
2. Data that goes into the LLM
3. LLM's output response
The `get_rag_eval_scores()` function returns a faithfulness score from 0 to 1.
"""
import sys
from textwrap import dedent
import pandas as pd
from lastmile_eval.rag import get_rag_eval_scores
from lastmile_eval.common.utils import get_lastmile_api_token
def main():
rag_scores_example_1()
rag_scores_example_2()
return 0
def rag_scores_example_1():
print("\n\nRAG scores example 1:")
statement1 = "the sky is red"
statement2 = "the sky is blue"
queries = ["what color is the sky?", "is the sky blue?"]
data = [statement1, statement1]
responses = [statement1, statement2]
api_token = get_lastmile_api_token()
result = get_rag_eval_scores(
queries,
data,
responses,
api_token,
)
print("Result: ", result)
# result will look something like:
# {'p_faithful': [0.9955534338951111, 6.857347034383565e-05]}
def rag_scores_example_2():
print("\n\nRAG scores example 2:")
questions = ["what is the ultimate purpose of the endpoint?"] * 2
data1 = """
Server-side, we will need to expose a prompt_schemas endpoint
which provides the mapping of model name → prompt schema
which we will use for rendering prompt input/settings/metadata on the client
"""
data = [data1] * 2
responses = ["""client rendering""", """metadata mapping"""]
# f"{data1}. Query: {questions[0]}",
# f"{data1}. Query: {questions[1]}",
print(f"Input batch:")
df = pd.DataFrame(
{"question": questions, "data": data, "response": responses}
)
print(df)
api_token = get_lastmile_api_token()
result_dict = get_rag_eval_scores(
questions,
data,
responses,
api_token,
)
df["p_faithful"] = result_dict["p_faithful"]
print(
dedent(
"""
Given a question and reference data (assumed to be factual),
the faithfulness score estimates whether
the response correctly answers the question according to the given data.
"""
)
)
print("Dataframe with scores:")
print(df)
if __name__ == "__main__":
sys.exit(main())
"""The text module provides more general evaluation functions
for text generated by AI models."""
import sys
import dotenv
import lastmile_eval.text as lm_eval_text
from lastmile_eval.common.utils import load_dotenv_from_cwd
def main():
# Openai evaluators require openai API key in .env file.
# See README.md for more information about `.env`.
load_dotenv_from_cwd()
SUPPORTED_BACKING_LLMS = [
"gpt-3.5-turbo",
"gpt-4",
]
print("Starting text evaluation examples.")
for model_name in SUPPORTED_BACKING_LLMS:
print(
f"\n\n\n\nRunning example evaluators with backing LLM {model_name}"
)
text_scores_example_1(model_name)
text_scores_example_2(model_name)
text_scores_example_3(model_name)
return 0
def text_scores_example_1(model_name: str):
texts_to_evaluate = [
"The quick brown fox jumps over the lazy dog.",
"The quick brown fox jumps over the lazy dog.",
]
references = [
"The quick brown fox jumps over the lazy dog.",
"The swift brown fox leaps over the lazy dog.",
]
bleu = lm_eval_text.calculate_bleu_score(texts_to_evaluate, references)
print("\n\nTexts to evaluate: ", texts_to_evaluate)
print("References: ", references)
print("\nBLEU scores: ", bleu)
rouge1 = lm_eval_text.calculate_rouge1_score(texts_to_evaluate, references)
print("\nROUGE1 scores: ", rouge1)
exact_match = lm_eval_text.calculate_exact_match_score(
texts_to_evaluate, references
)
print("\nExact match scores: ", exact_match)
relevance = lm_eval_text.calculate_relevance_score(
texts_to_evaluate, references, model_name=model_name
)
print("\nRelevance scores: ", relevance)
summarization = lm_eval_text.calculate_summarization_score(
texts_to_evaluate, references, model_name=model_name
)
print("\nSummarization scores: ", summarization)
custom_semantic_similarity = (
lm_eval_text.calculate_custom_llm_metric_example_semantic_similarity(
texts_to_evaluate, references, model_name=model_name
)
)
print("\nCustom semantic similarity scores: ", custom_semantic_similarity)
def text_scores_example_2(model_name: str):
texts_to_evaluate = [
"The quick brown fox jumps over the lazy dog.",
"The quick brown fox jumps over the lazy dog.",
]
references = [
"The quick brown fox jumps over the lazy dog.",
"The swift brown fox leaps over the lazy dog.",
]
questions = ["What does the animal do", "Describe the fox"]
qa = lm_eval_text.calculate_qa_score(
texts_to_evaluate, references, questions, model_name=model_name
)
print("\n\nTexts to evaluate: ", texts_to_evaluate)
print("References: ", references)
print("\nQA scores: ", qa)
def text_scores_example_3(model_name: str):
texts_to_evaluate = [
"I am happy",
"I am sad",
]
toxicity = lm_eval_text.calculate_toxicity_score(
texts_to_evaluate, model_name=model_name
)
print("\nToxicity scores: ", toxicity)
custom_sentiment = (
lm_eval_text.calculate_custom_llm_metric_example_sentiment(
texts_to_evaluate, model_name=model_name
)
)
print("\nCustom sentiment scores: ", custom_sentiment)
if __name__ == "__main__":
sys.exit(main())
FAQs
An API to measure evaluation criteria (ex: faithfulness) of generative AI outputs
We found that lastmile-eval demonstrated a healthy version release cadence and project activity because the last version was released less than a year ago. It has 5 open source maintainers collaborating on the project.
Did you know?
Socket for GitHub automatically highlights issues in each pull request and monitors the health of all your open source dependencies. Discover the contents of your packages and block harmful activity before you install or update your dependencies.
Security News
vlt's new "reproduce" tool verifies npm packages against their source code, outperforming traditional provenance adoption in the JavaScript ecosystem.
Research
Security News
Socket researchers uncovered a malicious PyPI package exploiting Deezer’s API to enable coordinated music piracy through API abuse and C2 server control.
Research
The Socket Research Team discovered a malicious npm package, '@ton-wallet/create', stealing cryptocurrency wallet keys from developers and users in the TON ecosystem.