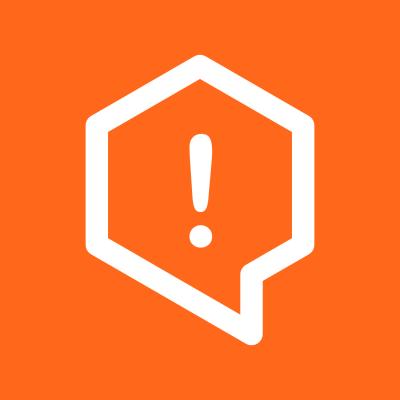
Security News
Fluent Assertions Faces Backlash After Abandoning Open Source Licensing
Fluent Assertions is facing backlash after dropping the Apache license for a commercial model, leaving users blindsided and questioning contributor rights.
llama-index-llms-ollama
Advanced tools
To install the required package, run:
pip install llama-index-llms-ollama
localhost:11434
.Ollama
instance by specifying model=":"
.Ollama(..., request_timeout=300.0)
.llm = Ollama(..., model="<model family>")
without a version, it will automatically look for the latest version.from llama_index.llms.ollama import Ollama
llm = Ollama(model="llama3.1:latest", request_timeout=120.0)
To generate a text completion for a prompt, use the complete
method:
resp = llm.complete("Who is Paul Graham?")
print(resp)
To send a chat message and receive a response, create a list of ChatMessage
instances and use the chat
method:
from llama_index.core.llms import ChatMessage
messages = [
ChatMessage(
role="system", content="You are a pirate with a colorful personality."
),
ChatMessage(role="user", content="What is your name?"),
]
resp = llm.chat(messages)
print(resp)
To stream responses for a prompt, use the stream_complete
method:
response = llm.stream_complete("Who is Paul Graham?")
for r in response:
print(r.delta, end="")
To stream chat responses, use the stream_chat
method:
messages = [
ChatMessage(
role="system", content="You are a pirate with a colorful personality."
),
ChatMessage(role="user", content="What is your name?"),
]
resp = llm.stream_chat(messages)
for r in resp:
print(r.delta, end="")
Ollama supports a JSON mode to ensure all responses are valid JSON, which is useful for tools that need to parse structured outputs:
llm = Ollama(model="llama3.1:latest", request_timeout=120.0, json_mode=True)
response = llm.complete(
"Who is Paul Graham? Output as a structured JSON object."
)
print(str(response))
You can attach a Pydantic class to the LLM to ensure structured outputs:
from llama_index.core.bridge.pydantic import BaseModel
from llama_index.core.tools import FunctionTool
class Song(BaseModel):
"""A song with name and artist."""
name: str
artist: str
llm = Ollama(model="llama3.1:latest", request_timeout=120.0)
sllm = llm.as_structured_llm(Song)
response = sllm.chat([ChatMessage(role="user", content="Name a random song!")])
print(
response.message.content
) # e.g., {"name": "Yesterday", "artist": "The Beatles"}
You can also use asynchronous chat:
response = await sllm.achat(
[ChatMessage(role="user", content="Name a random song!")]
)
print(response.message.content)
FAQs
llama-index llms ollama integration
We found that llama-index-llms-ollama demonstrated a healthy version release cadence and project activity because the last version was released less than a year ago. It has 1 open source maintainer collaborating on the project.
Did you know?
Socket for GitHub automatically highlights issues in each pull request and monitors the health of all your open source dependencies. Discover the contents of your packages and block harmful activity before you install or update your dependencies.
Security News
Fluent Assertions is facing backlash after dropping the Apache license for a commercial model, leaving users blindsided and questioning contributor rights.
Research
Security News
Socket researchers uncover the risks of a malicious Python package targeting Discord developers.
Security News
The UK is proposing a bold ban on ransomware payments by public entities to disrupt cybercrime, protect critical services, and lead global cybersecurity efforts.