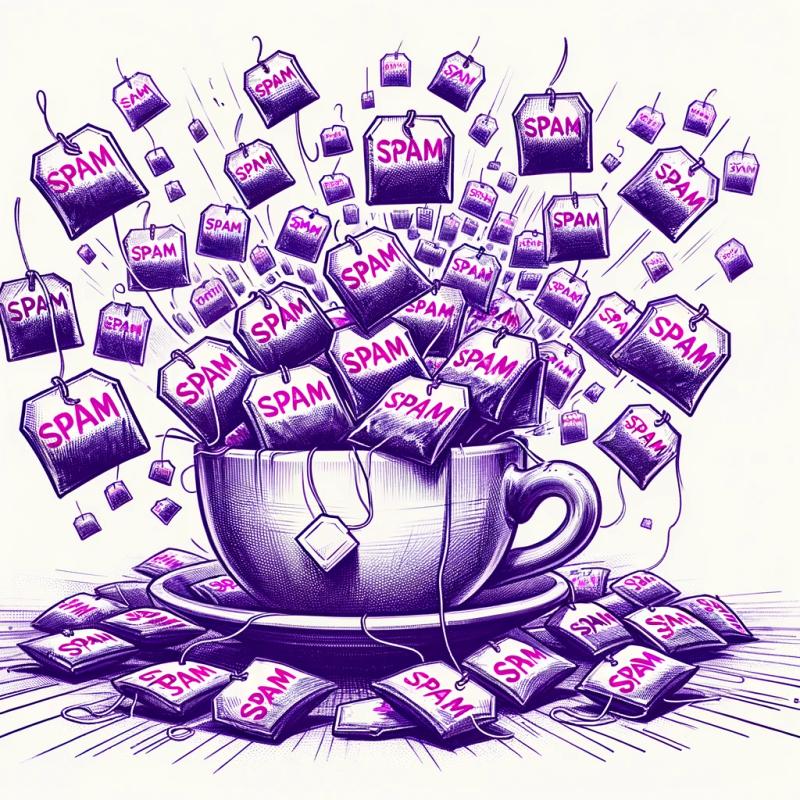
Security News
tea.xyz Spam Plagues npm and RubyGems Package Registries
Tea.xyz, a crypto project aimed at rewarding open source contributions, is once again facing backlash due to an influx of spam packages flooding public package registries.
Readme
This is a Python client for Replicate. It lets you run models from your Python code or Jupyter notebook, and do various other things on Replicate.
👋 Check out an interactive version of this tutorial on Google Colab.
pip install replicate
Before running any Python scripts that use the API, you need to set your Replicate API token in your environment.
Grab your token from replicate.com/account and set it as an environment variable:
export REPLICATE_API_TOKEN=<your token>
We recommend not adding the token directly to your source code, because you don't want to put your credentials in source control. If anyone used your API key, their usage would be charged to your account.
Create a new Python file and add the following code, replacing the model identifier and input with your own:
>>> import replicate
>>> replicate.run(
"stability-ai/stable-diffusion:27b93a2413e7f36cd83da926f3656280b2931564ff050bf9575f1fdf9bcd7478",
input={"prompt": "a 19th century portrait of a wombat gentleman"}
)
['https://replicate.com/api/models/stability-ai/stable-diffusion/files/50fcac81-865d-499e-81ac-49de0cb79264/out-0.png']
Some models, particularly language models, may not require the version string. Refer to the API documentation for the model for more on the specifics:
replicate.run(
"meta/llama-2-70b-chat",
input={
"prompt": "Can you write a poem about open source machine learning?",
"system_prompt": "You are a helpful, respectful and honest assistant.",
},
)
Some models, like andreasjansson/blip-2, have files as inputs. To run a model that takes a file input, pass a URL to a publicly accessible file. Or, for smaller files (<10MB), you can pass a file handle directly.
>>> output = replicate.run(
"andreasjansson/blip-2:f677695e5e89f8b236e52ecd1d3f01beb44c34606419bcc19345e046d8f786f9",
input={ "image": open("path/to/mystery.jpg") }
)
"an astronaut riding a horse"
[!NOTE] You can also use the Replicate client asynchronously by prepending
async_
to the method name.Here's an example of how to run several predictions concurrently and wait for them all to complete:
import asyncio import replicate # https://replicate.com/stability-ai/sdxl model_version = "stability-ai/sdxl:39ed52f2a78e934b3ba6e2a89f5b1c712de7dfea535525255b1aa35c5565e08b" prompts = [ f"A chariot pulled by a team of {count} rainbow unicorns" for count in ["two", "four", "six", "eight"] ] async with asyncio.TaskGroup() as tg: tasks = [ tg.create_task(replicate.async_run(model_version, input={"prompt": prompt})) for prompt in prompts ] results = await asyncio.gather(*tasks) print(results)
Replicate’s API supports server-sent event streams (SSEs) for language models.
Use the stream
method to consume tokens as they're produced by the model.
import replicate
# https://replicate.com/meta/llama-2-70b-chat
model_version = "meta/llama-2-70b-chat:02e509c789964a7ea8736978a43525956ef40397be9033abf9fd2badfe68c9e3"
for event in replicate.stream(
model_version,
input={
"prompt": "Please write a haiku about llamas.",
},
):
print(str(event), end="")
You can also stream the output of a prediction you create. This is helpful when you want the ID of the prediction separate from its output.
version = "02e509c789964a7ea8736978a43525956ef40397be9033abf9fd2badfe68c9e3"
prediction = replicate.predictions.create(
version=version,
input={"prompt": "Please write a haiku about llamas."},
stream=True,
)
for event in prediction.stream():
print(str(event), end="")
For more information, see "Streaming output" in Replicate's docs.
You can start a model and run it in the background:
>>> model = replicate.models.get("kvfrans/clipdraw")
>>> version = model.versions.get("5797a99edc939ea0e9242d5e8c9cb3bc7d125b1eac21bda852e5cb79ede2cd9b")
>>> prediction = replicate.predictions.create(
version=version,
input={"prompt":"Watercolor painting of an underwater submarine"})
>>> prediction
Prediction(...)
>>> prediction.status
'starting'
>>> dict(prediction)
{"id": "...", "status": "starting", ...}
>>> prediction.reload()
>>> prediction.status
'processing'
>>> print(prediction.logs)
iteration: 0, render:loss: -0.6171875
iteration: 10, render:loss: -0.92236328125
iteration: 20, render:loss: -1.197265625
iteration: 30, render:loss: -1.3994140625
>>> prediction.wait()
>>> prediction.status
'succeeded'
>>> prediction.output
'https://.../output.png'
You can run a model and get a webhook when it completes, instead of waiting for it to finish:
model = replicate.models.get("ai-forever/kandinsky-2.2")
version = model.versions.get("ea1addaab376f4dc227f5368bbd8eff901820fd1cc14ed8cad63b29249e9d463")
prediction = replicate.predictions.create(
version=version,
input={"prompt":"Watercolor painting of an underwater submarine"},
webhook="https://example.com/your-webhook",
webhook_events_filter=["completed"]
)
For details on receiving webhooks, see replicate.com/docs/webhooks.
You can run a model and feed the output into another model:
laionide = replicate.models.get("afiaka87/laionide-v4").versions.get("b21cbe271e65c1718f2999b038c18b45e21e4fba961181fbfae9342fc53b9e05")
swinir = replicate.models.get("jingyunliang/swinir").versions.get("660d922d33153019e8c263a3bba265de882e7f4f70396546b6c9c8f9d47a021a")
image = laionide.predict(prompt="avocado armchair")
upscaled_image = swinir.predict(image=image)
Run a model and get its output while it's running:
iterator = replicate.run(
"pixray/text2image:5c347a4bfa1d4523a58ae614c2194e15f2ae682b57e3797a5bb468920aa70ebf",
input={"prompts": "san francisco sunset"}
)
for image in iterator:
display(image)
You can cancel a running prediction:
>>> model = replicate.models.get("kvfrans/clipdraw")
>>> version = model.versions.get("5797a99edc939ea0e9242d5e8c9cb3bc7d125b1eac21bda852e5cb79ede2cd9b")
>>> prediction = replicate.predictions.create(
version=version,
input={"prompt":"Watercolor painting of an underwater submarine"}
)
>>> prediction.status
'starting'
>>> prediction.cancel()
>>> prediction.reload()
>>> prediction.status
'canceled'
You can list all the predictions you've run:
replicate.predictions.list()
# [<Prediction: 8b0ba5ab4d85>, <Prediction: 494900564e8c>]
Lists of predictions are paginated. You can get the next page of predictions by passing the next
property as an argument to the list
method:
page1 = replicate.predictions.list()
if page1.next:
page2 = replicate.predictions.list(page1.next)
Output files are returned as HTTPS URLs. You can load an output file as a buffer:
import replicate
from PIL import Image
from urllib.request import urlretrieve
out = replicate.run(
"stability-ai/stable-diffusion:27b93a2413e7f36cd83da926f3656280b2931564ff050bf9575f1fdf9bcd7478",
input={"prompt": "wavy colorful abstract patterns, oceans"}
)
urlretrieve(out[0], "/tmp/out.png")
background = Image.open("/tmp/out.png")
You can the models you've created:
replicate.models.list()
Lists of models are paginated. You can get the next page of models by passing the next
property as an argument to the list
method, or you can use the paginate
method to fetch pages automatically.
# Automatic pagination using `replicate.paginate` (recommended)
models = []
for page in replicate.paginate(replicate.models.list):
models.extend(page.results)
if len(models) > 100:
break
# Manual pagination using `next` cursors
page = replicate.models.list()
while page:
models.extend(page.results)
if len(models) > 100:
break
page = replicate.models.list(page.next) if page.next else None
You can also find collections of featured models on Replicate:
>>> collections = [collection for page in replicate.paginate(replicate.collections.list) for collection in page]
>>> collections[0].slug
"vision-models"
>>> collections[0].description
"Multimodal large language models with vision capabilities like object detection and optical character recognition (OCR)"
>>> replicate.collections.get("text-to-image").models
[<Model: stability-ai/sdxl>, ...]
You can create a model for a user or organization with a given name, visibility, and hardware SKU:
import replicate
model = replicate.models.create(
owner="your-username",
name="my-model",
visibility="public",
hardware="gpu-a40-large"
)
Here's how to list of all the available hardware for running models on Replicate:
>>> [hw.sku for hw in replicate.hardware.list()]
['cpu', 'gpu-t4', 'gpu-a40-small', 'gpu-a40-large']
Use the training API to fine-tune models to make them better at a particular task. To see what language models currently support fine-tuning, check out Replicate's collection of trainable language models.
If you're looking to fine-tune image models, check out Replicate's guide to fine-tuning image models.
Here's how to fine-tune a model on Replicate:
training = replicate.trainings.create(
model="stability-ai/sdxl",
version="39ed52f2a78e934b3ba6e2a89f5b1c712de7dfea535525255b1aa35c5565e08b",
input={
"input_images": "https://my-domain/training-images.zip",
"token_string": "TOK",
"caption_prefix": "a photo of TOK",
"max_train_steps": 1000,
"use_face_detection_instead": False
},
# You need to create a model on Replicate that will be the destination for the trained version.
destination="your-username/model-name"
)
See CONTRIBUTING.md
FAQs
Python client for Replicate
We found that replicate demonstrated a healthy version release cadence and project activity because the last version was released less than a year ago. It has 3 open source maintainers collaborating on the project.
Did you know?
Socket for GitHub automatically highlights issues in each pull request and monitors the health of all your open source dependencies. Discover the contents of your packages and block harmful activity before you install or update your dependencies.
Security News
Tea.xyz, a crypto project aimed at rewarding open source contributions, is once again facing backlash due to an influx of spam packages flooding public package registries.
Security News
As cyber threats become more autonomous, AI-powered defenses are crucial for businesses to stay ahead of attackers who can exploit software vulnerabilities at scale.
Security News
UnitedHealth Group disclosed that the ransomware attack on Change Healthcare compromised protected health information for millions in the U.S., with estimated costs to the company expected to reach $1 billion.